Dr. Shanshan Wang is an associate professor at Shenzhen Institutes of Advanced Technology, Chinese Academy of Sciences. She received her dual Ph.D degree from the University of Sydney and Shanghai Jiaotong University respectively in information technologies and biomedical engineering. Dr. Wang was a finalist for the Australian John Makepeace Bennett Best Thesis Award and won the 2018 OCSMRM Outstanding Research Award. Her research interests include machine learning, fast medical imaging and biomedical signal analysis.
Below is a summary of her presentation
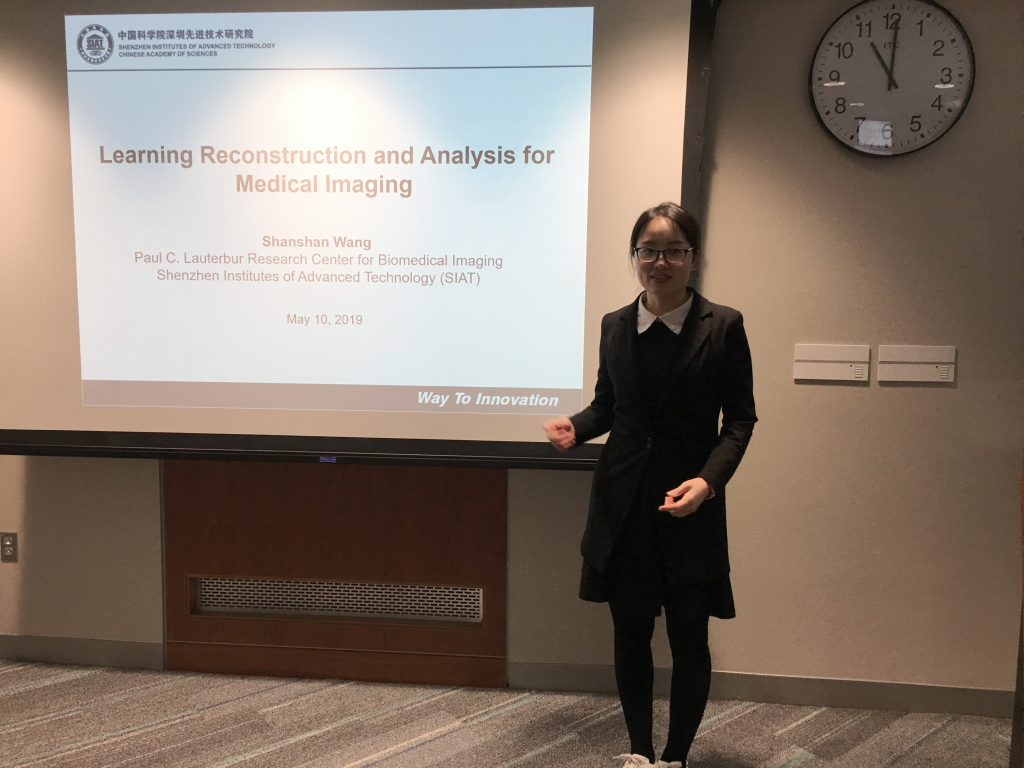
Inverse problems are ubiquitous in the field of medical imaging and image processing. Prominent examples include fast MR imaging and image denoising. The goal of these problems is to reconstruct or restore an unknown image from a set of direct/indirect measurements. However, due to the limitation of the acquisition time or the existence of noise, the obtained measurements are often corrupted or incomplete, which introduces big challenges for the reconstruction process and the following clinical diagnosis. In order to remove the noise or overcome the ill-posed nature caused by the insufficient measurements, it is necessary to explore the prior knowledge and utilize them to form constraints in the reconstruction process so as to make up for the missing or corrupted information.
However, traditional prior knowledge regularizations and their corresponding algorithms suffer from loss of the detailed information such as texture and structure while reducing the image degradation factors. To attack these issues, Dr. Wang and her colleagues have developed a series of machine learning based medical imaging methods and algorithms, which can draw valuable prior from either the target or offline medical images and therefore assist efficient clinical uses. To better facilitate clinical diagnosis, they also have sought to extract quantitative features from medical images to uncover disease characteristics that fail to be appreciated by the naked eye. They try to build computer aided tools to release the clinicians from boring and tedious work like image segmentation, classification and lesion detection. The developed methods are being applied in different clinical settings including stroke MR imaging using deep prior learning, dictionary learning for undersampled reconstruction, as well as breast cancer analysis with neural network guided lesion classification and segmentation algorithms.