Leslie Ying is currently a Professor of Biomedical Engineering and Electrical Engineering at the University at Buffalo, SUNY. She received her B.E. in Electronics Engineering from Tsinghua University, China in 1997 and both her M.S. and Ph.D. in Electrical Engineering from the University of Illinois at Urbana – Champaign in 1999 and 2003, respectively.
Below is a summary of her presentation
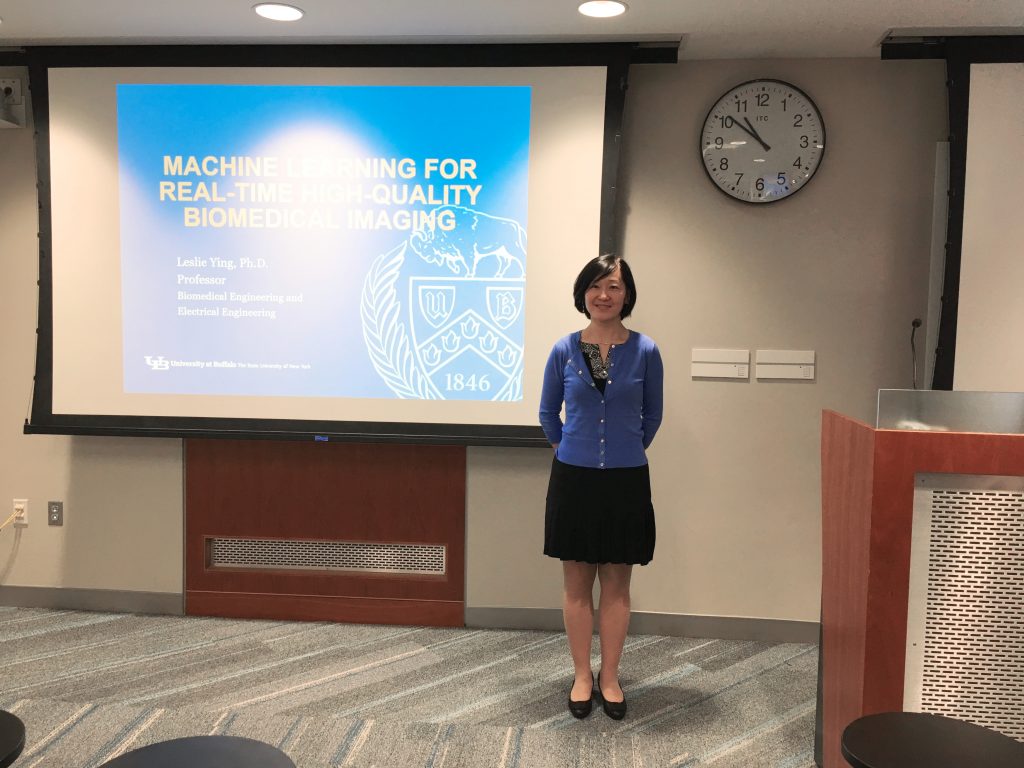
Machine learning has recently attracted a lot of attention in biomedical imaging. It has shown success in biomedical image classifications but only very recently been used for image reconstruction with unique features. For this talk, Dr. Ying started with compressed sensing (CS), a strategy for reconstruction from sub-Nyquist sampled data. Several machine-learning-based methods were introduced within the conventional CS framework. She then explained how the optimization algorithm underlying CS can be unrolled to a deep artificial neural network, such that parameters and prior models can be learned from training samples. Finally, end-to-end convolutional neural networks were presented based on the training data with little knowledge of the imaging system. Connections among different networks were discussed with their benefits and limitations highlighted. Although most examples provided were from MRI, the frameworks are generalizable to image reconstruction problems for most imaging modalities. The talk concluded with future outlooks.