Dr. Chen is a Professor of Medical Physics and Radiology at the Univ. of Wisconsin-Madison. He received his BS degree in physics from Beijing Normal Univ. in 1991, a Master’s Degree in mathematics from Nankai Univ. in 1995, and a PhD in semiconductor physics from the Univ. of Utah in 2000. He published extensively in a variety of physics research topics such as quantum computing, spintronics, quantum optics, semiconductor, superconductor, quantum field theory, and high energy string theory. In 2002, he shifted his research interests to medical physics to apply his knowledge of both physics and mathematics to conduct innovative research in x-ray computed tomography (CT) imaging.
Below is a summary of his talk
Pushing the boundaries of human intelligence to enable next generation CT imaging technology
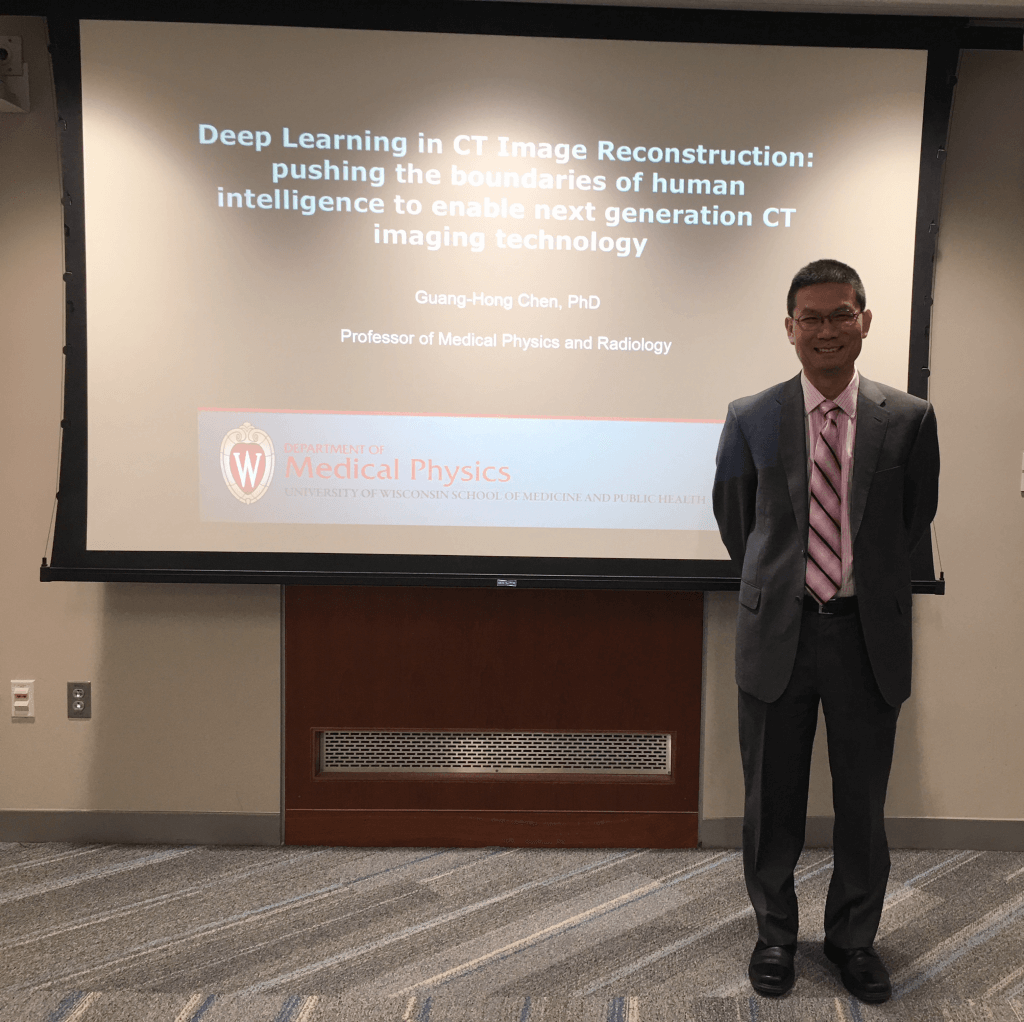
Given the tremendous improvements in computational power offered by graphics processing units (GPUs) and other dedicated computation units, deep neural network architectures have been successfully trained to produce impressive results in computer vision, gaming, natural language processing, image processing, and recently, medical imaging. In this talk, Dr. Chen discussed how deep learning methods can be leveraged to address several challenging CT image reconstruction problems providing excellent empirical generalizability, and the application of these methods in current and future CT imaging. He then discussed the potential paradigm shift in CT image reconstruction enabled by deep learning methods. To conclude, he discussed the potential challenges and opportunities in applying deep learning to CT imaging.