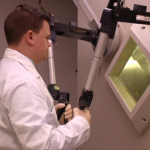
Radiopharmaceutical company Trace-Ability, Inc. announced that the FDA has approved the first use of its solution, Tracer-QC, for release testing Ammonia N-13 Injection at the MGH Gordon Center PET Core. Tracer-QC automates the positron emission tomography (PET) tracer release testing process, which the company says is known for its complexity. “Despite the clear value of […]